VR4CH: visual recognition services for the cultural heritage sector
What can online visual recognition services (VRS) do for cultural heritage image collections? In 2018, we investigated this together with content partner MoMu (Fashion Museum of Antwerp), and IT research and consultancy company, Datable.
Challenge
The manual registration of museum objects, archives and other cultural heritage collections is a labour-intensive process, generally limited to purely administrative and formal data. Substantive metadata are not always available, even though this is often what’s required to make collections searchable. We set up an exploratory research project together with MoMu and Datable to see if we could fill this gap in a time- and cost-efficient way. Artificial intelligence (AI) is a very promising avenue to explore here, and we wanted to find out whether the organisations in the cultural heritage sector could use automated software to help with their registration processes.
Even though image recognition software is already used to unlock commercial image databases, it’s still quite rare in the cultural heritage community. These applications are often not feasible or usable for heritage organisations because they don’t have the resources and expertise they need to implement these services in their day-to-day collection management processes.
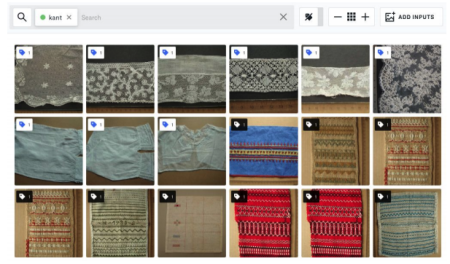
Result of upload on a model that was trained to distinguish between lace and samplers © Clarifai
Aim
With support from Datable and meemoo, MoMu wants to deploy visual recognition services in order to find out to what extent museums can describe and unlock their image collections better and more efficiently without any big investments.
Our rol
Meemoo supported this exploratory research and pilot project with its expertise, and facilitated the applicability of the project in the heritage sector. We set up the pilot project with image content from MoMu’s research and museum collections, and the visual recognition services were given the task of providing 164 images with description tags. Some of the images had already been assigned basic information such as title, content and object name, which meant we were able to compare this information with the results from our exercise.
The VR4CH (Visual Recognition for Cultural Heritage) project ran from 2018 to 2019 and was made possible thanks to an Innovative Partner Projects grant from the Flemish Community (only in Dutch).
Approach
Visual recognition technology is already offered as ‘Software as a Service’ (SaaS) on some platforms. We chose to test and compare Microsoft Computer Vision, Google Cloud Vision and Clarifai in this project, because these service providers offer a suitably extensive platform, a relatively simple API and good documentation. They are also cloud-based VRS applications which you can start using without any further configuration or training. You can also make a number of free calls via these platforms, whereby you send the image in question to the VRS and receive an answer in the form of description tags. This makes them more accessible for organisations with limited resources that simply want to experiment with the technology.
Conclusion
The strengths of VRS:
VRS is faster and cheaper than manual, human registration;
The technology is particularly good at providing images with basic descriptions;
The intricacy of the descriptions is unexpectedly high – VRS can describe different parts of an object separately, so the results are certainly good enough to provide heritage collections with indicative, global descriptions;
It also covers objects and categories which generally fall outside the conventional scope of registration, such as colour and genre (e.g. ‘fashion’ or ‘glamour’).
Visual recognition services score significantly lower than human registration for more specialist descriptions, however, because they lack the technical and historical knowledge and context that domain experts possess for correctly describing objects in detail based on just a single image.
Depending on requirements and expectations, it’s definitely worth trying out VRS. These services often produce sufficiently usable results if a certain margin of error is acceptable. Furthermore, you can combine the speed of visual recognition services with the precision of human registration. This approach can help institutions that manage collections, use their time and resources efficiently, and so reduce any backlog they might have.
When using image recognition technology, it’s important to work with models that are best suited to the image content and expected outcomes, and also to set a threshold to reduce the number of mistakes based on the description tags’ probability score. Results can be further improved by:
manually checking the description tags;
automatic filtering to remove unwanted or irrelevant tags;
working with clusters to categorise images by content or visual characteristics, and/or verify them with a keyword list to enrich the data.
>> Read the full report on CEST (only in Dutch)